Why Differentiation Matters in the Next Wave of Generative AI
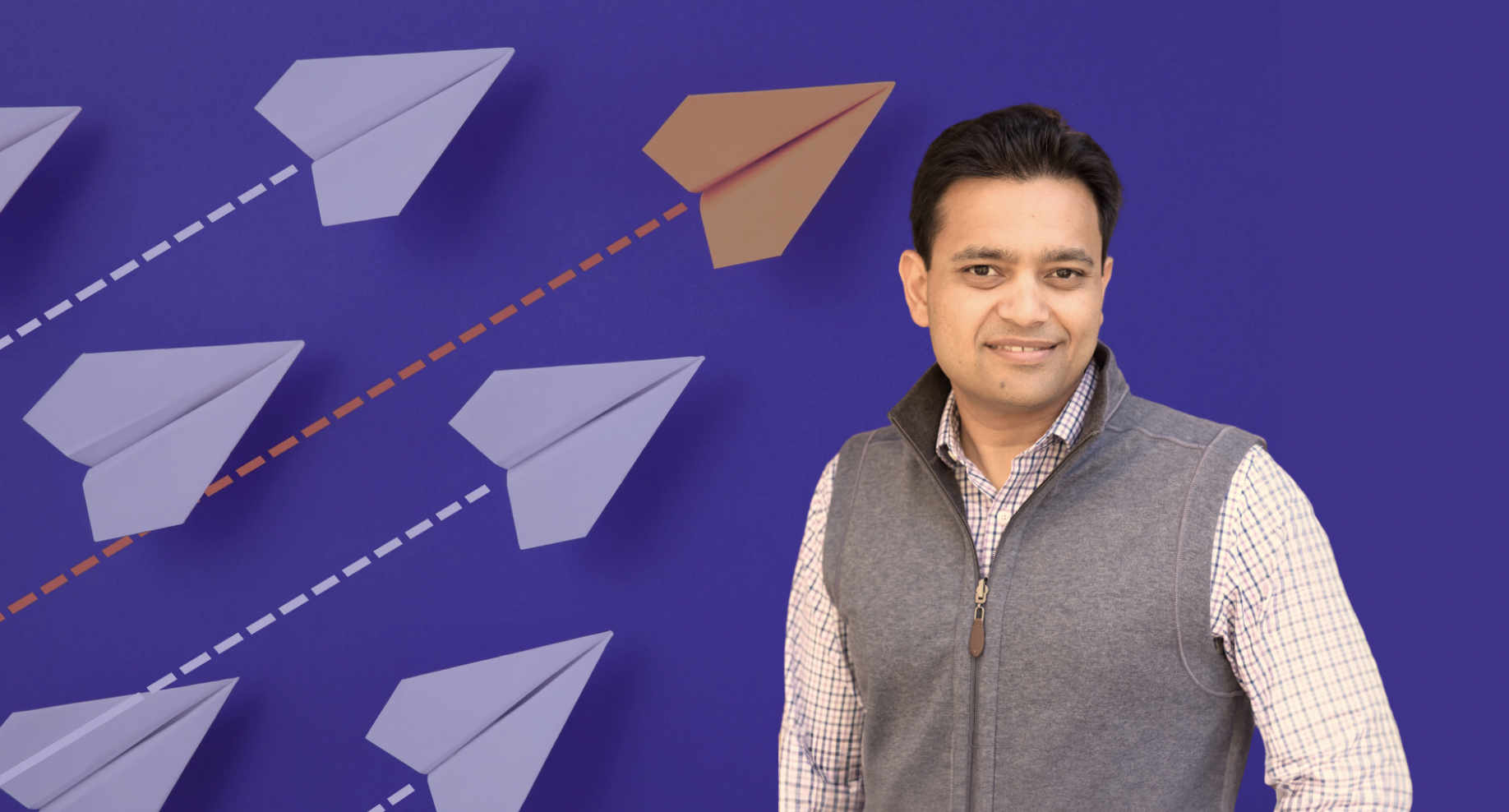
Over the past year, I transitioned from being a full-time operator—working at companies like Workday, Kroger, and Citi, and founding VNDLY (acquired by Workday)—to becoming a Managing Partner at Sierra Ventures, where we have a rich history of investing in AI. The evolution of the AI market, particularly with generative AI (GenAI), has been remarkable.
GenAI reminds me of the trajectory of blockchain and Bitcoin. Initially, the focus was on Bitcoin’s value, then shifted to blockchain’s technology, and eventually circled back to Bitcoin. Similarly, AI has evolved from general AI to robotic process automation (RPA) and now to GenAI, with large language models (LLMs) emerging as transformative value drivers. These LLMs are revolutionizing knowledge work, turning massive data into actionable insights and fundamentally reshaping enterprise operations.
From an investment standpoint, the next 12–18 months will determine whether general-purpose GenAI tools or domain-specific AI agents dominate. Startups excel in agility and focus but often lack the scale of training data that big tech wields. Conversely, tech giants may struggle with the depth and customization startups offer. The critical question: Will enterprises adopt horizontal solutions from big tech, or will they prefer specialized, startup-driven innovations?
Compelling GenAI use cases are already shifting from workflow automation to outcome-driven solutions. For instance, our portfolio company Pantomath integrates with ServiceNow to automate the creation of detailed tickets pinpointing data issues. This innovation slashes resolution times by 50–70%, directly impacting operational efficiency. Similarly, companies like Siena, CICERO AI, and Sedai are pioneering agentic AI for domains like customer service, legal tech, and cloud cost management.
Big tech is also advancing in agentic AI. Salesforce’s Agentforce, Workday, and ServiceNow are integrating AI across their platforms, but these solutions remain horizontal, leaving room for startups to carve niches with deeper specialization. The challenge for GenAI startups is building defensible platforms rather than features that big tech could replicate.
The broader implications of LLMs are profound. We’re moving from SaaS (Software as a Service) to “Services as a Software,” disrupting traditional business process outsourcing. By encoding repetitive, knowledge-based tasks into LLMs, enterprises can transform cost structures, driving productivity gains that outpace RPA’s impact.
However, challenges persist. At Sierra’s 19th annual CXO Summit, CIOs highlighted hurdles like data quality, privacy, and infrastructure. Enterprises are laying the groundwork, and in the next 2–3 years, improved data hygiene and governance will unlock GenAI’s potential. Meanwhile, we’re investing in AI-driven security solutions, such as ArmorCode and Weav.AI, which address emerging needs for enterprises deciding whether to build or lease their LLMs.
When evaluating early-stage GenAI startups, one question guides us: Is this a feature or a platform? Startups must articulate a clear differentiation and a path to becoming indispensable platforms. My advice to founders: Define your unique value, ensure your defensibility, and demonstrate how your solution scales beyond its initial use case. In a crowded market, clarity and vision are paramount.