AI Agents: Revolutionizing Enterprise Decision-Making and Complex Task Automation
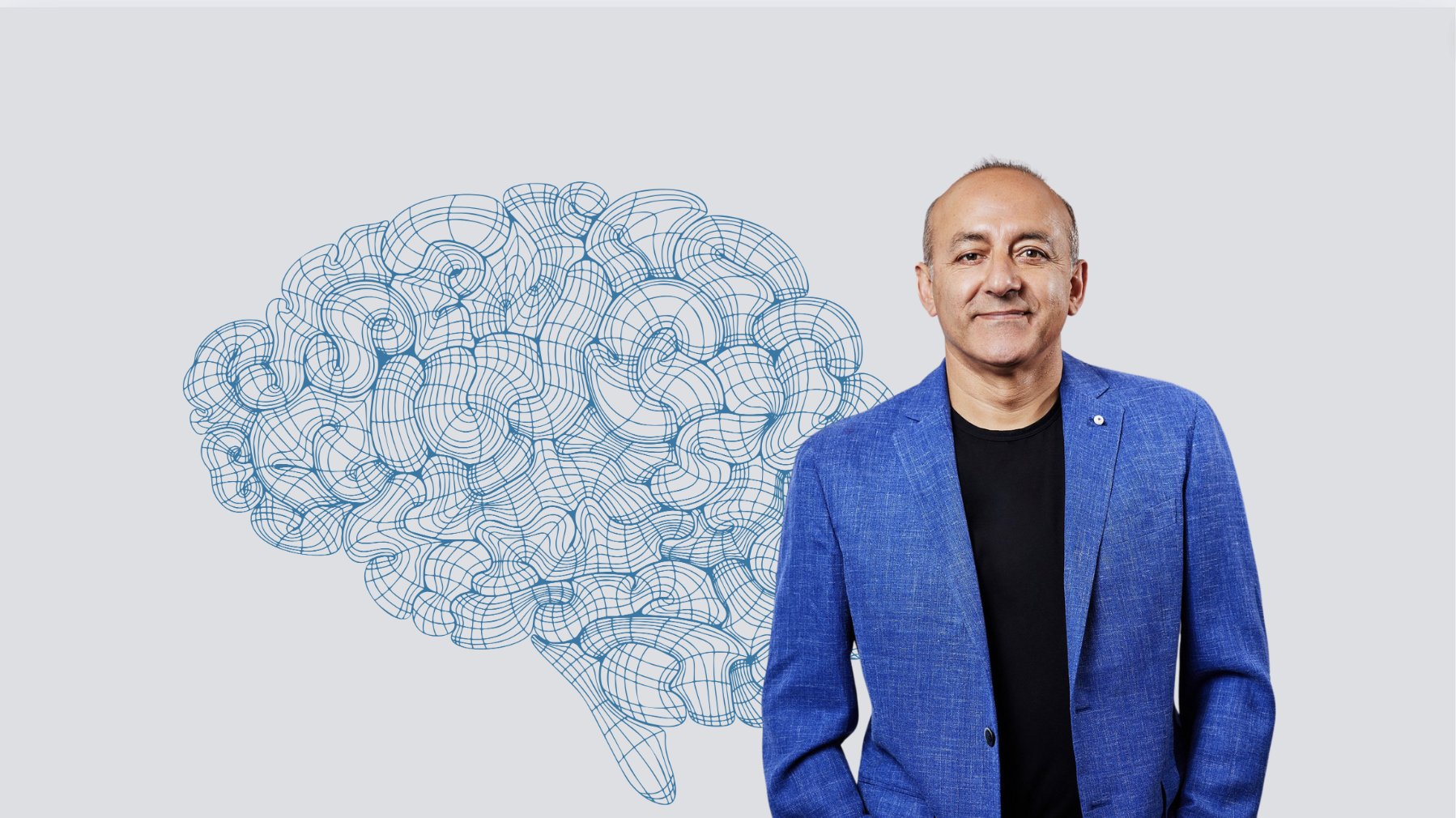
Agents represent the next step in the evolution of AI applications and the deployment of Machine Learning. They are compound systems of one or more AI models with advanced reasoning abilities and access to software tools they use to make decisions and perform complex tasks. They combine the ability of AI models to understand and respond more closely to how people communicate (through natural language, visual, and auditory means) with the efficiency and reliability of software and can do so at scale.
There are multiple advantages to building and deploying agent architectures instead of monolithic AI applications. They can:
- Take over broader pieces of work from humans and abstract away repetitive tasks with a larger scope
- Combine multiple modalities (e.g., text, audio, visual, 3D) or specialized models into one single agent
- Provide more reliable mathematical capabilities than State-of-the-Art LLMs
- Increase the accuracy of responses by including helper models that fact-check and provide Quality Assurance for the rest of the agent
- Preserve data privacy by isolating sensitive information processing to a dedicated, specially designed model
- Manage inference costs by only running some of the smaller models in the system, as needed, instead of one large one
- Provide better observability into agent behavior and the ability to fine-tune individual components to manage performance and risk
Al Agents in the Enterprise: From RAG to Autonomy
The potential of Agentic systems in the Enterprise is tremendous. They will be deployed across all industries to improve customer interactions, from sales to support, as well as all major business functions.
This potential will be fully realized when existing product and service providers in all major sectors deliver significantly more reliable, accurate, and faster customer experiences. They will be able to do so because AI agents will make complex decisions and perform key business functions at scale with superior accuracy and speed.
Industry-Specific Example: In the insurance industry, underwriters and claims adjusters rely on manual methods and personal judgment to assess risks and evaluate claims. This approach is time-consuming and error-prone and can lead to inconsistent decisions, reducing efficiency and customer satisfaction. Insurance enterprise copilots can use single agentic AI as the primary generative AI capability. Copilots can be leveraged for Underwriting, Claims, and Insurance Product development, using AI agents to perform complex analyses like exposure, loss, and claims analyses. Our portfolio company, weav.ai is tackling this solution. Their agents connect to multiple information sources and proprietary ML models to collect all the necessary information, triangulate it, and recommend a decision based on current and historical data. These agents not only reduce the cost of underwriting and claims by 70% —90% but, more importantly, enable the underwriters and claims adjusters to make more accurate decisions, thus improving their profitability. Working with their design partners, they are tracking to deliver over 60M of value over a three-year period.
|
Agents Will Evolve From Retrieval Augmented Generation to Multi-Agent Systems that Handle Broader, More Complex Decisions and Tasks
Agents are starting out as slightly more complex systems than stand-alone AI models and, with time, will take on larger, more intricate tasks. They will also be able to integrate into more proprietary and customized systems as they become more specialized and business leaders gain trust in these systems.
The first generation of AI agents are Retrieval Augmented Generation applications, which use a search index and engine assigned to a data repository (e.g. the web, enterprise warehouses) alongside a LLM in order to provide answers that are grounded in existing documents.
More advanced agents promise to be able to make decisions about the tools they use, plan the workflow, gather the information or access the resources that they need from other computer systems or people, execute the task, and return the completed work to the user.
These advanced capabilities will allow companies to delegate complex business decisions and scale key tasks (i.e., customer support) to provide exceptional user experiences at a lower cost. When deploying agents across multiple corporate functions with various seniority and autonomy levels, these systems will collaborate, thus creating Multi-Agent networks that can make decisions on behalf of and take care of entire business functions.
Example: Cloud management within organizations continues to be a problem. Specialized AI agents focusing on different aspects of operations—like cost, uptime, and deployment—are a solution to optimize cloud operations. Our portfolio company, Sedai, has built this solution. Sedai an AI-powered autonomous cloud management platform that continuously optimizes cloud operations by detecting, prioritizing, and analyzing performance metrics. Its agents work across finance (cost), operations (uptime), and engineering (new releases) to optimize uptime while keeping cost the lowest, thereby enabling cloud teams to maximize cost savings, performance, and availability. Their agents have enabled shifting from static rules and threshold-based automation to modern, ML-based autonomous operations, allowing teams to focus more on innovation. Customers are realizing 30%+ cost savings while scaling infrastructure. |
The impact of AI agents in the enterprise is not only to cut costs, but also to enable firms to reroute capital into building even stronger differentiation and innovation for their products and services, as well as allow employees to scale down the amount of repetitive decisions and tasks in their job in favor of even more complex and creative responsibilities.
Evolution of AI Agents in the Enterprise
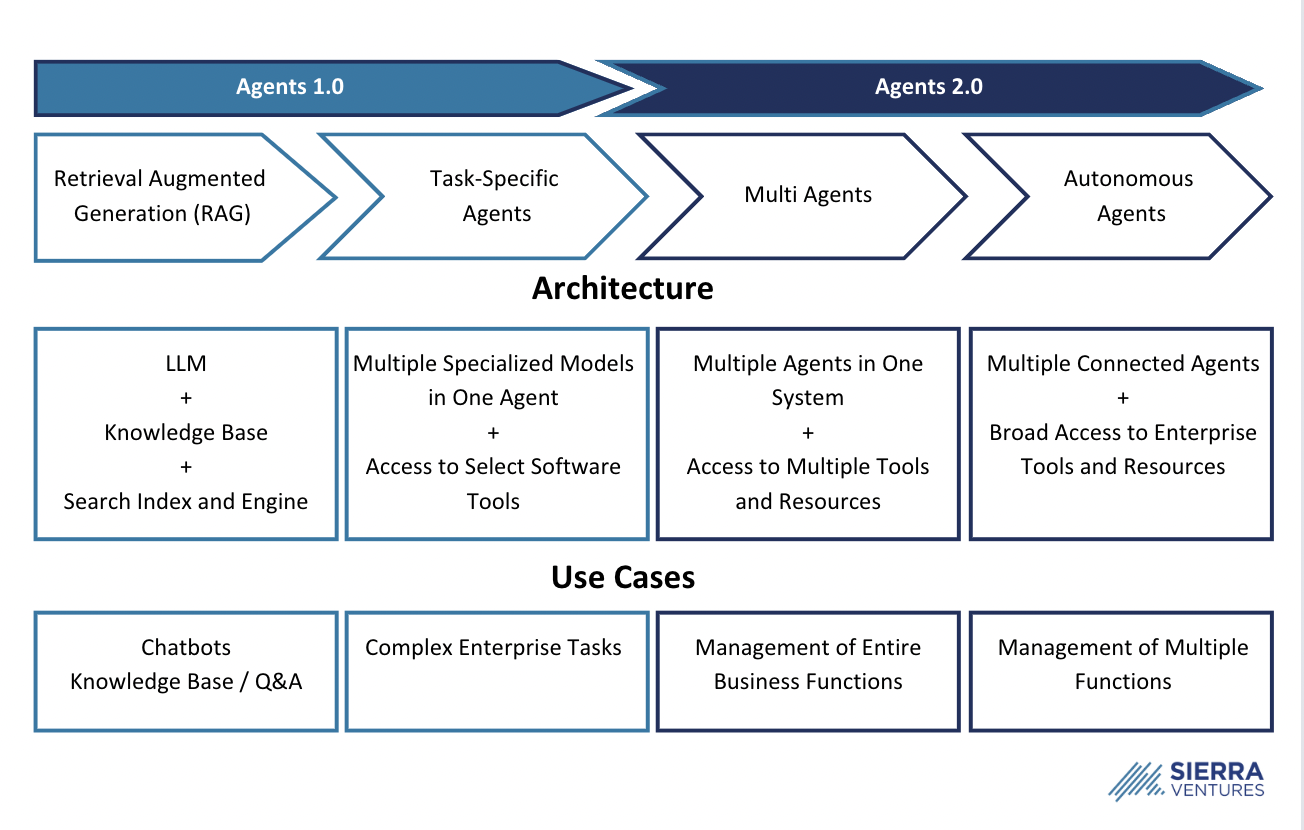
Besides RAG systems developed for enterprise search and Q&A applications, some of the advanced agent architectures for enterprise include:
- A LLM with function calling abilities, combined with a calculator, in order to perform well in tasks that require mathematical reasoning for applications such as finance and accounting
- A system made up of multiple smaller, specialized models that are coordinated and supervised by a principal, larger model for applications that require domain-specific knowledge, such as healthcare, science and legal.
- A LLM that has access to a browser and adjacent productivity tools, such as a text editor or sketchpad, for applications involving information retrieval and processing from the web, such as competitive research and market analysis
- One or more LLMs that perform a main task, combined with several Quality Assurance and Security & Privacy models that ensure that no sensitive information is inadvertently released and that the answers provided to the user are accurate
- Multi-agent systems comprised of teams of agents with various specializations, which are activated and used only when needed, based on the requirements of the task they are employed for
Vertical Agentic SaaS Automation Provides the Largest Opportunity and Moat for AI Start-up Founders
There is tremendous potential for Vertical AI Agents in the Enterprise because they have been underserved in the past. This is mostly because of the deep context needed to operate in those domains.
Vertical agents' key strength is getting trained and optimized for the specific processes within an industry that require deep context and specialization. While the volume of one process might not be enormous when compared to horizontal applications, the value that it provides will be strategically very large, thus creating strong product engagement and competitive advantage for both the Agent provider and customer base. Most importantly, these verticals are ‘green field’, i.e., they have never been automated before and, therefore, represent the most efficient way to scale software companies in the future.
The addressable market for vertical agents will consist of the following:
- SMBs who want to make their operations more cost-effective and/or create stronger differentiation for their products
- Large Enterprises who want to expand their offering to perform more complex work and reduce their workforce footprint.
- Agencies providing specialized services, such as creative, legal, recruiting, and financial services firms, will sell “output” or “work” done by AI agents to better and faster serve their customers.
- Aggregators and marketplaces of agents, who will enable the discovery and supply of a wide variety of agentic systems
If you are a start-up founder building AI agents for the Enterprise, here are important considerations to shape your strategy:
- Vertical agentic SaaS automation provides great opportunities, especially for open, pluggable systems that integrate seamlessly with customers’ processes and operations.
- Distribution within your target vertical will be key, so building your customer network and focusing on system integrators at a strategic level will drive good engagement from the beginning. SI’s don’t have the expertise to build agentic systems, but have the market reach.
- An open architecture will prevent vendor or AI lock-in for your customers and create engagement driven by the value and user delight you create. It will also harmonize working collaboratively with IT efforts in Gen AI and ensure they are not detractors but supporters.
- Setting specific agent performance metrics and benchmarks for your use case and vertical will be important as you define your product strategy and focus on the type of value to provide to your customers
AI agents are poised to transform enterprise operations by handling complex tasks and making informed decisions at scale. As businesses integrate these agents, they can focus more on innovation and creativity, while the agents manage routine tasks and optimize performance across key functions.