A Tectonic Shift of Generative AI Adoption in the Enterprise
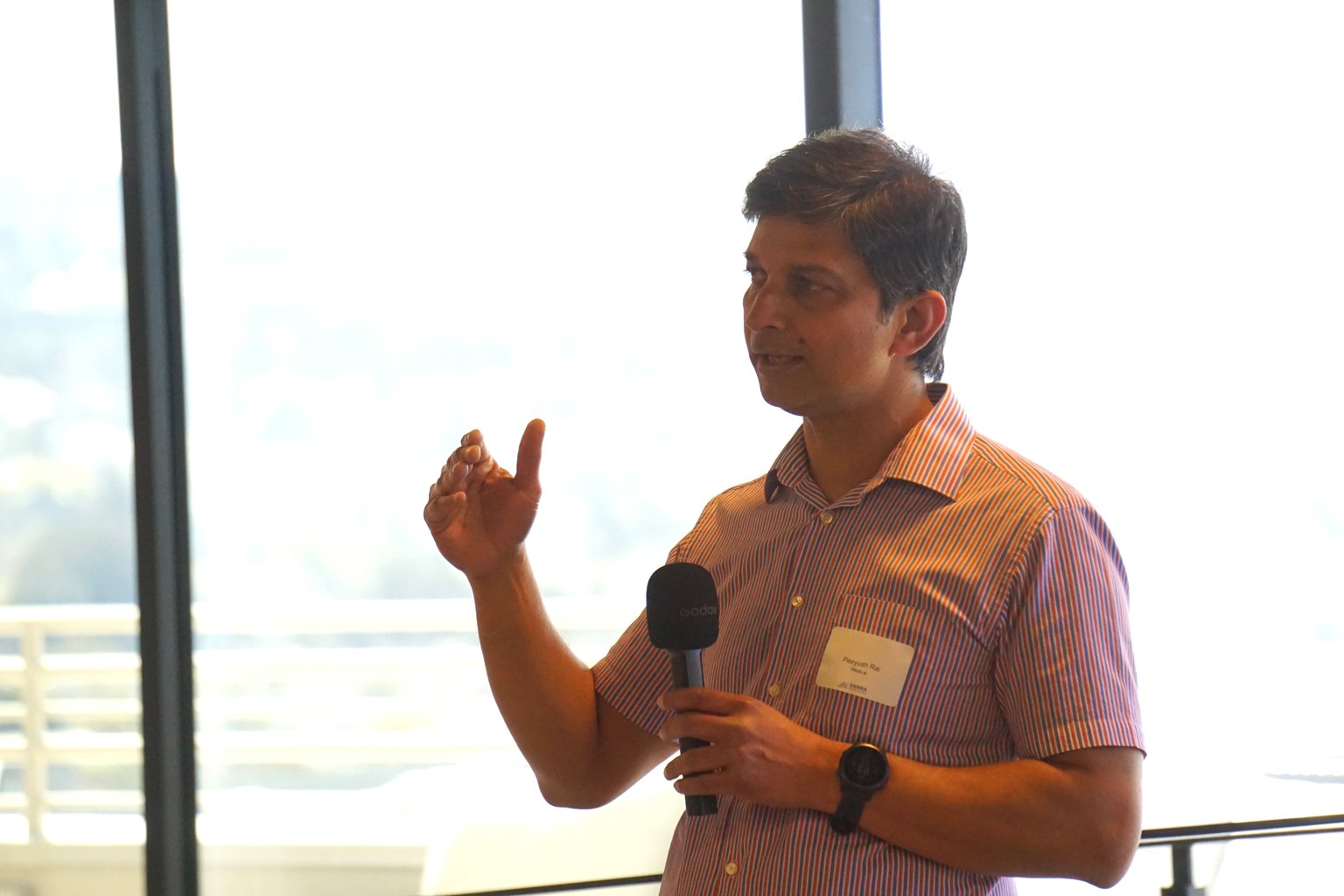
Generative AI is a subset of AI that focuses on creating new content rather than simply classifying or predicting existing data. It works by analyzing patterns and trends in large amounts of data and using that knowledge to create new content.
At the recent “Generative AI in the Enterprise'' conference put together by Sierra Ventures, a number of generative AI experts gathered to share their insights. Peeyush Rai, CEO of weav.ai, opened the event with an interesting talk on the status of generative AI today and how the adoption of this technology is undergoing a tectonic shift. If enterprises utilize generative AI to the greatest effect, there are a few critical things to remember.
Although generative AI has existed for several years, recent applications built on Large Language Models like BERT and GPT3/GPT4 have made these tools more accessible and prolific in the business world. Following the popularity of DallE and ChatGPT with the general public, individuals and companies are increasingly recognizing the various applications of generative AI — and making it happen. A new dawn seems to have broken, offering a radical reimagining of how we get things done.
Generative AI: Definition & Origin
By definition, generative AI is a technology that can comprehend human input and generate a response in the form of text, images, audio, or video. It produces these responses by identifying patterns in the large volumes of data it has been trained on; it doesn’t use spontaneous thought or logic to come to an answer but instead selects the most likely response based on the existing data it has looked at. By using these trends and learnings in combination, generative AI can create new content out of existing material.
The data these models are trained on are usually a large corpus of labeled data. This can be open datasets available on the internet or domain-specific data. The models trained with this technique are called foundation models. By their very nature, the more inputs these algorithms are fed, the more advanced they will grow. This means they can also be applied to use case-specific purposes; now that several of the models have been made available to the public, it is possible for an organization to take a database like BERT or DallE and then train it on a specific domain. For instance, Rai highlighted Bloomberg’s recent project to retrain a foundational model specifically for financial applications, which required a substantial investment of 53 days and millions of dollars — but one that is still more accessible than in previous years.
Key Use Cases for the Enterprise
Within the enterprise, the applications are tenfold, but specific functions are most suited to generative AI’s capabilities.
Marketing & Personalization
A company can create its own generative AI application fed with information about its customer base, product, etc. This algorithm will then generate recommendations solely from its own catalog of offerings that are then tailored solely to its audience. Further segmentation allows teams to identify appropriate personalization for specific groups within this audience for a more individual experience — all achieved more easily than existing technology supports.
Customer Support
Current iterations of conversational AI have improved the chatbot experience drastically, yet their limitations still cause many customers to request a human agent to resolve their issues. With more advanced generative AI, these chat assistants can take over a majority of simple customer support requests, as they will understand a greater range of queries and be able to offer more nuanced responses. This will free up human agents for more complex issues and allow businesses to keep their costs down without compromising service.
Coding Assistance for IT Teams
Generative AI cannot produce truly original thought, but it can draw from many existing resources and greatly support creative coders within an enterprise. Particularly if the AI is trained on that company’s IP, software developers can teach their algorithm their unique codes and build a database of knowledge only accessible by their own employees. This will speed up the time it takes to develop new applications, providing developers with the support they need to focus on the creative aspect of their work.
HR & Operations
Many solutions scan resumes for keywords and simplify the hiring process, but these limited tools can eliminate strong candidates if not optimized properly. A well-trained generative AI algorithm can be trained on a company’s specific ethos and hiring preferences for tailored recommendations. For existing employees, AI can also become a more engaging source of training and resources, adaptable to each team’s needs. Operations teams can leverage the database for more detailed analytics and predictive maintenance.
The Requirements for Building Generative AI Apps
The rewards are clear, but organizations need specific infrastructure, tools, and skills to build generative AI applications. Although many innovation-minded companies may already have comprehensive development teams and even machine learning teams, this new generation of AI needs a new paradigm of development, and companies must invest accordingly.
Specifically, Rai recommends that more businesses consider investing in GPUs which, although in existence, are currently underutilized but will be very valuable for generative AI. Also, on the infrastructure side, vector databases will be critical for text and image functions. From a security perspective, the risks are greater when dealing with a language model without sufficient context or protections. Dedicated prompt security and generative AI governance & compliance are critical for overall safety.
To achieve this, AI management will also be a critical component, whether context management, prompt design or system guard rails. These features will help companies maintain a secure working environment for their apps past the initial deployment point. As these applications expand and evolve, organizations will want to consider a dedicated focus on generative AI APIs and models. Once all these systems are in place, there will be an appropriate environment to focus on launching generative AI apps from chatbots to content generation.
What to Watch Out For
The opportunities are great, but these early stages of implementation mean there are also a lot of unknowns. Enterprise organizations should move cautiously when introducing generative AI into their operations to minimize the risk of negative outcomes. Most importantly, users must be cognizant of the fact that this AI is a language-based technology that does not think for itself. Therefore, it can produce falsehoods and inaccuracies just as easily as the truth, simply due to its interpretation of the input or the database. Fact-checking outputs is critical if companies are to spot these “hallucinations” before they cause damage.
Additionally, generative AI can be used for negative purposes if bad actors take advantage. The technology is neither ‘good’ nor ‘bad;’ it simply responds to the prompts. Creating safeguards within the algorithm is highly recommended to reduce the risk of harmful outputs. This is also a good way to keep early use cases at the enterprise level within a more contained environment so that teams can really learn the nuances of how best to utilize the technology before launching at a larger scale.
The Key Stages of Implementation
The enterprises that choose to launch generative AI applications would be wise to follow these steps laid out by Rai. First, be critical of whether generative AI is the appropriate solution for this business problem; At the same time, the technology provides a lot of value, but it is not always the right choice, and existing machine learning may be more than sufficient. Next, invest in the right team with the specific skills needed for the application’s architecture and language processing. From there, identify the appropriate platform for your application — which may be a collaboration of frameworks and tools.
Organizations must be mindful of building governance and testing into each stage of development. As discussed, the security risks are high, so governance cannot be an afterthought. Once the application is fully designed, it’s time to launch it as a beta program and get valuable feedback from trusted sources before it’s rolled out at scale. This ensures there is a trust established for the final production stage.